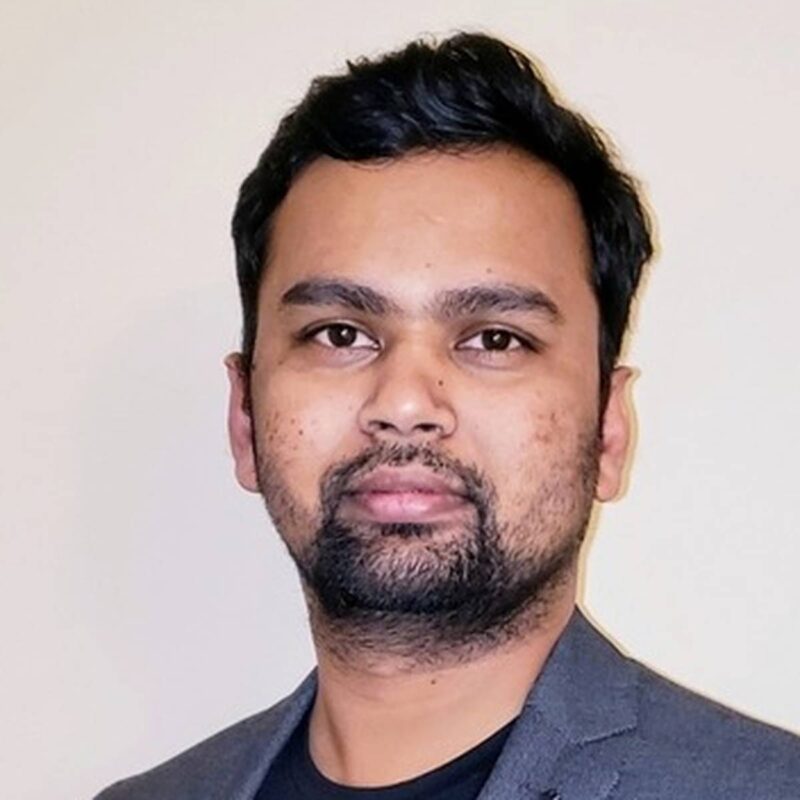
Speakers:
Data Science for the Manufacturing Supply chain
Date:
Thursday, June 6, 2024
Time:
10:30 am
Room:
Phoenix Ballroom A
Summary:
Data science is becoming increasingly relevant in the field of manufacturing, primarily in the areas of Materials Requirement Planning (MRP), Master Production Schedule (MPS) and Distribution Requirements Planning (DRP). This abstract focuses on the application and influence of AI and data science in these areas. Data science leverages statistical models, machine learning, forecasting and optimization to analyze and interpret complex data sets. In the manufacturing context, these techniques can be used to optimize operations, predict demand, and improve supply chain management. Specifically, MRP can utilize data science to optimize Raw material inventory levels, reduce lead times, and anticipate material requirements from downstream operations. MPS can leverage data science to enhance production scheduling and planning, minimize downtime, and improve capacity planning. DRP can benefit from data science by improving the accuracy of distribution plans, reducing stockouts, and minimizing holding costs. The integration of data science into MRP, MPS and DRP can lead to more efficient and effective manufacturing processes, thus enabling companies to gain a competitive edge in today’s data-driven business environment. However, the successful implementation of data science in manufacturing requires strong data infrastructure, skilled personnel, and a culture that encourages data-driven decision making.
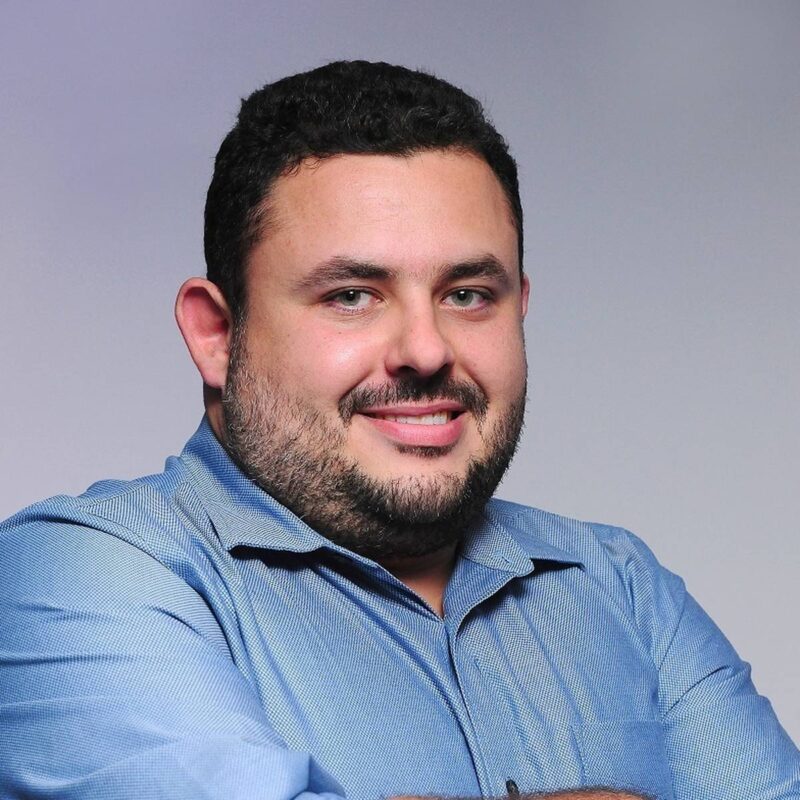
Speakers:
How Top5 Pharma Latam Captured Double-Digit Growth with ML and Analytics
Date:
Thursday, June 6, 2024
Time:
10:55 am
Summary:
This case study presents how Sanofi, a Global Top5 Pharma, bolstered its sales in LatAm with leads scoring and a recommendation model.
The Top5 Pharma Latin America was facing challenges to capture market share and increase sales force efficiency. The company decided to address this important challenge using analytics, which proved to be a successful approach. There were three main pitfalls to successfully pursue this agenda: their vision of analytics was highly technical, lacking business insights; the data lake was not fully operational; and data was siloed, spread out and with questionable quality.
The approach taken was to let the Business Challenge drive the team and the evolution on the technical side. To this end, the project analytically redesigned the daily agenda of sales reps, focusing on improving the relationship quality with physicians (who compose the main channel for market share capture in the industry). The team combined techniques, using regression and unsupervised learning, to structure the recommendation model (NBA). A second approach was a Lead Scoring model built with oversampling techniques and random forests.
In parallel, the analytics tech operation was evolved by structuring the data lake architecture and governance (real-time quality control on integration, data catalog implementation, etc.) and conducting new data sources integration to enable the use cases build. With business, data and tech on track, the team conducted the evolutionary backlog of both new business use cases, as well as lake and data sources modernization and integration.
The results were impressive: double digit prescription share growth in the target areas on the business side and data much more ready to use for the science and engineering teams.