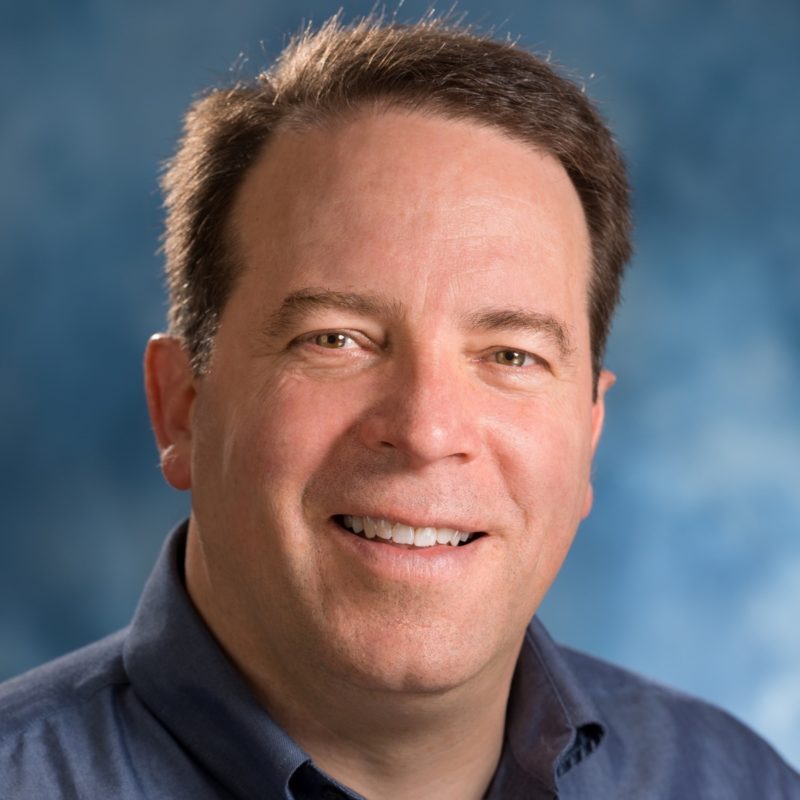
Speakers:
Special Plenary: The Reliability of Backpropagation is Worse than You Think
Date:
Friday, June 7, 2024
Time:
1:15 pm
Room:
Phoenix Ballroom D
Summary:
Neural Networks are flexible, powerful, and often very competitive in accuracy. They are criticized primarily for being uninterpretable black boxes, but their chief weakness is that backpropagation makes them unrepeatable. That is, their final coefficient values will differ, from one run to the next, even if the NN structure, meta-parameters, and data are held constant! And unlike multi-colinear regressions, the varied NN coefficient sets aren’t just alternative ways — in an over-parameterized model — of producing similar predictions. Instead, the predictions can vary a disquieting amount and often “converge” to a significantly worse training fit than is possible.
What happens if one instead employs a global optimization algorithm to train a NN? Untapped descriptive power should be unleashed, encouraging use of simpler structures to avoid overfit. And, with randomness removed, the results will be repeatable. We’ll demonstrate initial results for (the relatively small) NNs practical to optimize.