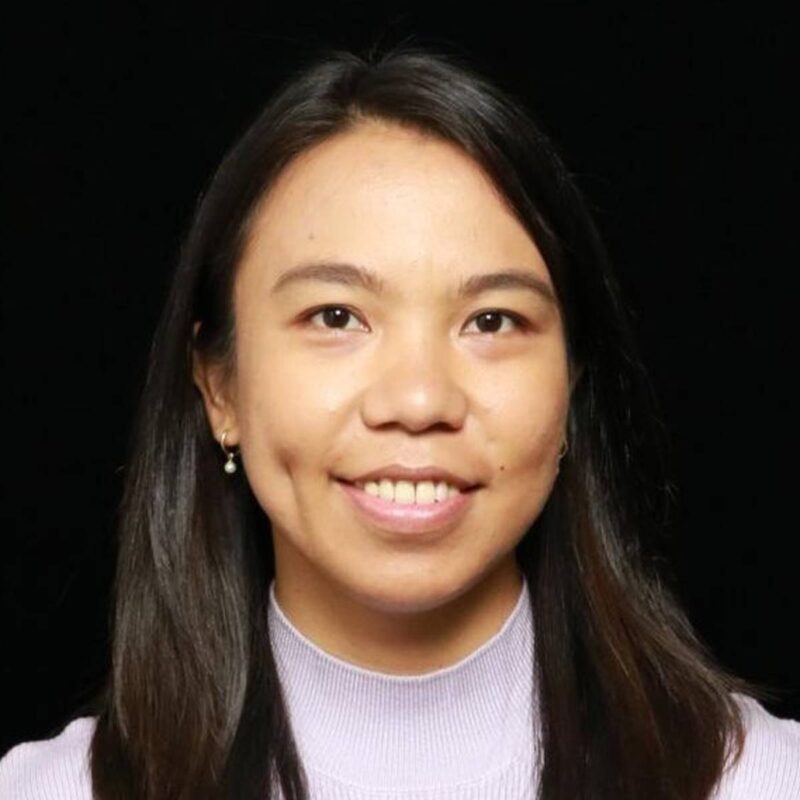
Speakers:
A Living Digital Twin of EV Batteries Using a Scalable Predictive Modeling Framework
Date:
Tuesday, June 20, 2023
Time:
2:15 pm
Track:
Room:
Red Rock Ballroom B
Summary:
We illustrate a Living Digital Twin of a fleet of Electric Vehicles that gives actionable predictions of battery degradation over time. Since each vehicle takes a different route and has different charging and discharging cycles over its lifetime, the battery degradation for each vehicle will be different. We use a scalable predictive modeling framework deployed across a distributed computing architecture in the cloud to make individualized predictions for each EV battery in the fleet to reflect the degraded performance accurately. The neural network battery model is calibrated using the parameters that had the most significant impact on the output voltage through the Unscented Kalman Filter (UKF) method, which is a Bayesian technique for parameter estimation of non-linear system behavior. We simulated in-production real-world operations by having the vehicles “drive” the routes using synthetic datasets and showed how the calibrated model provide more accurate estimates of battery degradation. Using a Living Digital Twin to calculate the remaining range and battery State of Health addresses problems of range anxiety in the EV automotive industry and can drive the value of EVs in the market.